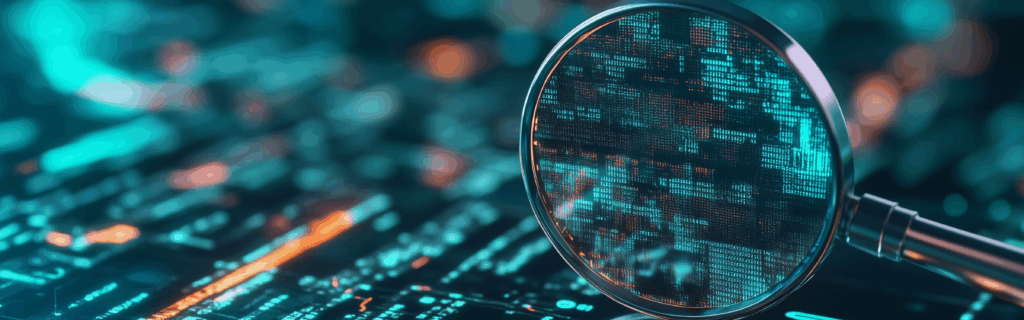
Share this post
To address the money mule problem, organisations must combine elements of fraud prevention, cyber threat intelligence, and anti-money laundering capabilities.
Is AML a real-time problem?
The answer seems to be “no” at first glance. Most money laundering typologies, such as transaction layering, rapid and high-frequency fund movements, and unusual counterparty relationships, require historical transaction data to identify suspicious patterns. It takes AML teams weeks (if not months) of diligent analysis to escalate these activities to law enforcement.
Money mules: A real-time AML problem demanding a real-time solution
However, money mule typologies present an opportunity to incorporate technology from fraud prevention, cyber threat intelligence, and AML to stop, investigate, and escalate illicit activity in real time.
Given the significant overlap between fraud and muling—both involving rapid, low-denomination fund movements through fraudulently onboarded or compromised accounts—fraud prevention tools can be applied to detect mule behaviour instantly. Additionally, unusual device, session, and account activity, often present in muling schemes, can be flagged by cyber threat intelligence tools to support immediate detection.
Yet, money mules aren’t just a fraud or cyber problem. They’re an essential part of the money laundering chain, placing and layering illicit funds through real-time payment systems. Under traditional AML programs, these small, rapid transactions often go unnoticed until enough activity accumulates to trigger a red flag, which is too late to stop the money laundering network. By then, the funds had been laundered through multiple accounts, making recovery difficult. This has significant impacts: in the UK alone, mules launder over £10 billion annually, with over 39,000 accounts demonstrating muling behaviour identified in 2022.
Mule detection: The convergence of fraud prevention, cyber threat intelligence, and AML
To dismantle mule networks faster, AML, fraud, and cyber teams must collaborate by sharing tools, data, and intelligence.
Fraud Prevention solutions have honed AI-based capabilities, including supervised machine learning (ML), which can be applied to mules to detect illicit transactions in real time. In addition, cyber threat intelligence tools enable real-time detection of accounts, credentials, and devices, providing supporting evidence to halt mule transfers in real-time, even without historical transaction patterns. Combined, these capabilities allow AML teams to act faster, stopping mule transactions in real time and using network analytics to uncover broader connections.
Here’s an example of this convergence:
- A small incoming transfer is scored as high risk by the fraud prevention system.
- Cyber threat tools detect unusual IP, device, and session activity.
- The transaction is halted in real time, and the account is flagged.
- AML systems identify a pattern of low-value incoming and outgoing transfers tied to multiple institutions.
- Network analytics identify connections to other mule accounts.
- Additional outgoing transfers are stopped, and a Suspicious Activity Report (SAR) is filed within the regulatory deadline.
Challenges in real-time AML
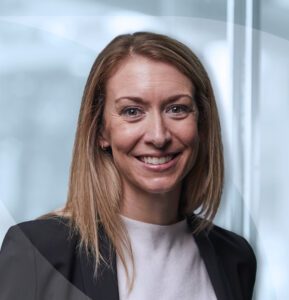
Real-time AML is easier said than done, given the current tooling and structure of most AML operations. Without automation, AI models, and integrated fraud and cyber threat signals, false positives can overwhelm AML teams. Institutions also need a way to quickly connect related transactions and accounts, ideally using graph analytics to map networks based on shared characteristics such as IP addresses, devices, or locations. Lastly, with a 30-day deadline for SAR filings once suspicious activity is detected, case management workflows and data collection need to be as streamlined and automated as possible.
Take action to address money mules
AML leaders should investigate whether their institution has a money mule problem and, if so, must prepare their operations for real-time AML:
- Perform a threat assessment of your portfolio: Investigate money mule typologies and patterns within your products, services, transactions, and accounts to determine your money mule risk exposure.
- Prepare your data for supervised ML. Advanced fraud prevention solutions use supervised ML to detect financial crimes accurately and provide critical signals for real-time AML. Accurately label transaction data as either fraud or genuine to prepare it for use in supervised models.
- Empower analysts and promote explainability with actionable context: Supplement real-time alerts with easily digestible intelligence to ensure explainability and help analysts make decisions. This includes cyber threat intelligence details and graph analytics that link alerting transactions to other customers flagged as exhibiting mule characteristics.
- Ensure your AML operations are ready for real-time alerts: Update AML processes and procedures by automating aspects of data collection and investigations, ensuring that analysts can quickly handle real-time alerts and submit suspicious activity reports (SARs) within regulatory timelines.
Conclusion
Money mules are a growing real-time AML problem that won’t be solved with siloed efforts. By merging the strengths of fraud prevention, cyber threat intelligence, and AML, institutions can detect, disrupt, and dismantle mule networks before laundered funds disappear.