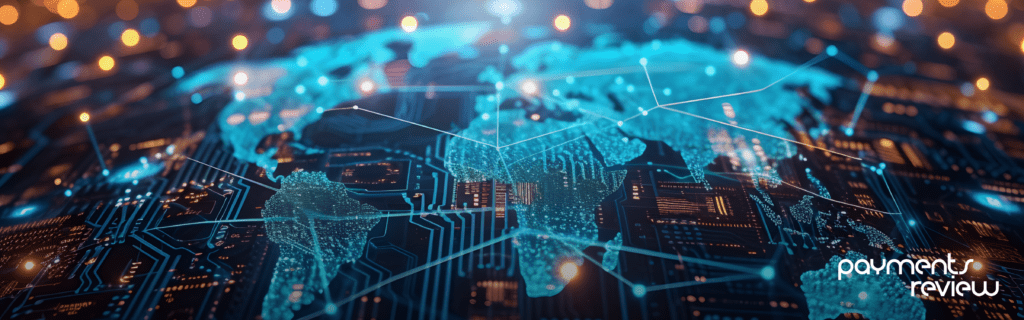
Share this post
In this Q&A, NVIDIA’s EMEA Payments & FinTech Leader, Georgios Kolovos, explains how AI is revolutionising fraud detection, risk management, and customer engagement in the payments industry.
How do you see AI fundamentally reshaping the payments landscape, particularly in fraud detection, risk management, and customer experience?
Georgios Kolovos: Payment companies see AI as crucial for future success if used strategically. From banks to digital wallets, AI adoption is expanding. Initially, focused on areas where machine learning and traditional AI played a key role, like fraud prevention and know your customer (KYC) and anti-money laundering (AML) compliance. Now, generative and agentic AI initiatives are becoming more sophisticated, enabling holistic customer engagement and boosting efficiency and merchant profitability.
What are the biggest AI-driven innovations helping financial institutions balance speed and security?
GK: AI algorithms, running on an accelerated computing platform, can analyse massive transaction data streams in real time. Enabled by parallel processing, it facilitates the identification of subtle anomalies and suspicious patterns indicative of fraud as transactions occur. This prevents fraudulent activity before it’s completed, rather than reacting after the damage is done. Think of it as having thousands of eyes scrutinising every transaction simultaneously. Fraudsters are increasingly using AI for sophisticated attacks.
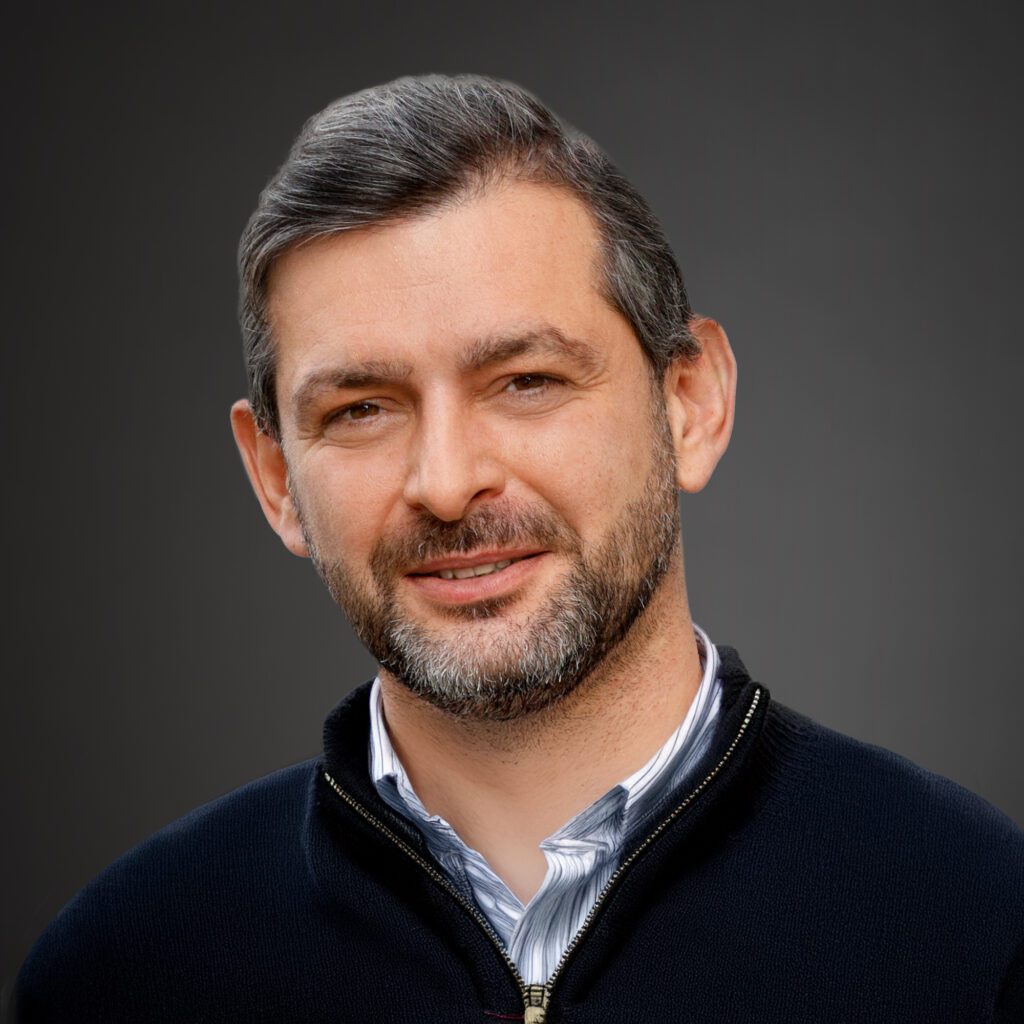
What strategies should financial institutions adopt to stay ahead in this AI arms race?
GK: Graph neural networks use transformers to identify complex relationships crucial for detecting sophisticated schemes. The scale and complexity of these models necessitate accelerated computing. Graphics processing units (GPUs) also provide the parallel processing power for model training and real-time fraud detection, ensuring effective prevention. Beyond the efforts of each organisation training new models with speed and accuracy, joining forces together via federated learning is an area which can enable
collaborative model training across entities without sharing sensitive data. False positives in fraud detection remain a major challenge for payments companies.
How can AI help reduce these errors without increasing risk?
GK: Traditional transaction monitoring relies on manually defined rules to flag suspicious activity, leading to high false positives and scalability issues. Using supervised and unsupervised learning, AIpowered systems automate fraud detection for greater accuracy and scalability. These models adapt quickly to evolving fraud tactics, continuously learning and reducing false positives. This benefits customers, merchants, and the payments ecosystem by reducing friction and building trust.
With regulators closely watching AI in financial services, how can the payments industry ensure compliance while leveraging AI’s full potential?
GK: AI offers a promising solution for AML, enabling computer systems to perform tasks autonomously by ingesting and analysing massive datasets, recognising complex patterns, and working with human oversight. This collaboration frees scarce human resources to focus on more complex investigations. AI, for example, streamlines suspicious activity reports (SARs). Just as payments companies leverage technologies today within existing regulatory frameworks, the same approach applies to AI, taking advantage of existing guardrails and expertise to address concerns like explainability and maintain alignment with regulatory requirements.
What role does AI play in improving KYC, AML, and identity verification processes for payments providers?
GK: AI models are designed to detect anomalies while tapping into additional unstructured data to identify the patterns, to make the process more efficient, identify subtle connections and patterns. Furthermore, users can act on the data in near-real time, enabling authorities to stay in step with the movements of bad actors, rather than remaining days or weeks behind. Generative AI can identify subtle connections and patterns that might have been missed, leading to more accurate risk assessments and improved AML compliance.